Messenger Lecture | “Differential Privacy and the US Census”
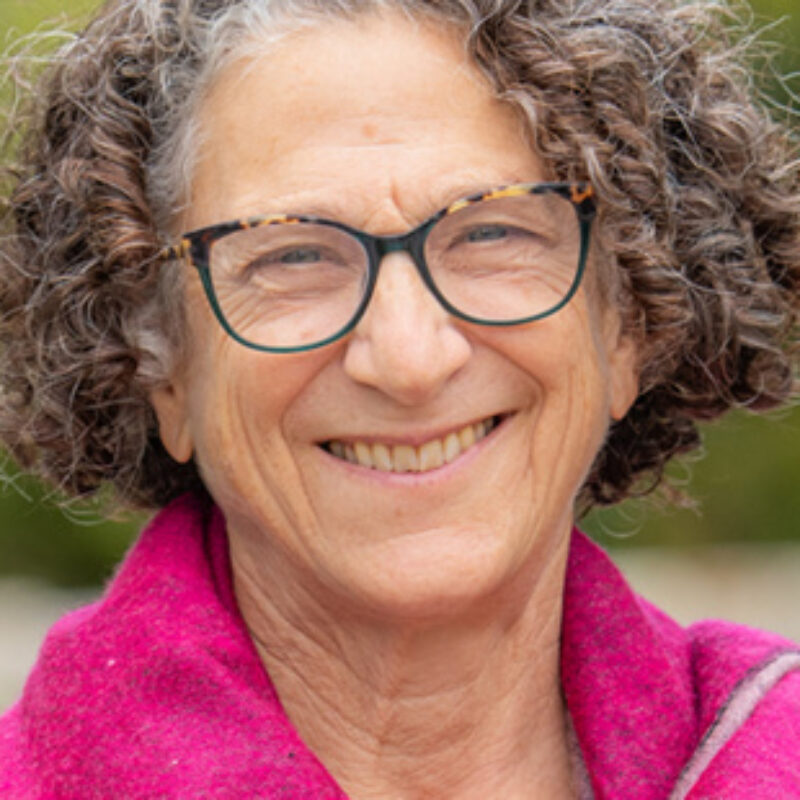
Cynthia Dwork is the Gordon McKay Professor of Computer Science at the Harvard University John A. Paulson School of Engineering and Applied Sciences and Affiliated Faculty at Harvard Law School.
She uses theoretical computer science to place societal problems on a firm mathematical foundation.
Her awards and honors include the National Medal of Science, the IEEE Hamming Medal, the RSA Award for Excellence in Mathematics, the Dijkstra, Gödel, and Knuth Prizes, and the ACM Paris Kanellakis Theory and Practice Award. She is a member of the US National Academy of Sciences and the US National Academy of Engineering, and is a Fellow of the American Academy of Arts and Sciences and the American Philosophical Society.
Abstract: Anonymized data aren’t: either they are not really anonymized or the anonymization process destroys their utility. Aggregate statistics, too, can fail to protect privacy, sometimes spectacularly. Predictive models trained on large datasets memorize substantial portions of the training data and have been tricked into revealing this information. The US Census Bureau demonstrated a privacy attack against the statistics the Bureau itself published in the 2010 census. Although there is provably(!) no magic bullet, Differential Privacy – a definition of privacy tailored to statistical data analysis and a collection of supporting algorithmic techniques — has proven fruitful in a wide range of settings, from generating QuickType suggestions on phones and computers to publication of US Census redistricting data.
Why is privacy so slippery? Why was this a new problem? What is Differential Privacy and how can be achieved? What happened when Alabama sued to prevent its deployment in the 2020 redistricting data?